- Medical AI Digest
- Posts
- AI Revolutionizing Sepsis Detection: Early Diagnosis Saves Lives
AI Revolutionizing Sepsis Detection: Early Diagnosis Saves Lives
Discover how AI leverages machine learning to detect sepsis early, reduce ICU mortality, and transform critical care with predictive analytics.
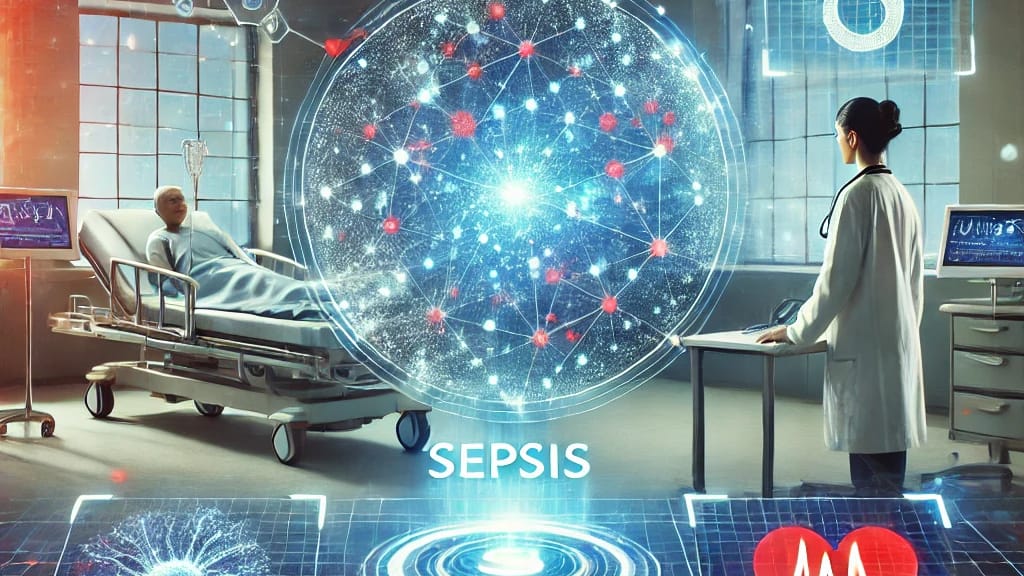
Sepsis, a life-threatening condition affecting over 1.7 million adults annually in the U.S. and leading to nearly 270,000 deaths, occurs when the body's response to infection results in tissue damage, organ failure, or potentially death. It remains a prominent cause of mortality in intensive care units (ICUs) worldwide. Despite advancements in critical care, early detection and treatment of sepsis continue to pose significant challenges for healthcare professionals. In this context, artificial intelligence (AI) emerges as a transformative tool that revolutionizes the approach to managing this deadly condition.
The Challenge of Sepsis Detection
Sepsis poses significant challenges in terms of early detection due to its often subtle initial symptoms and the potential for rapid, unpredictable progression. Traditional detection methods primarily utilize clinical scoring systems and biomarkers; however, these approaches frequently lack the precision and expeditiousness required for timely clinical interventions. Studies indicate that traditional methods can delay diagnosis by several hours, significantly elevating mortality risk, thereby underscoring the importance of early identification to save lives.
How AI Identifies Subtle Patterns in Vital Signs
AI-powered systems demonstrate exceptional capability in analyzing extensive volumes of patient data, effectively identifying patterns that may surpass human observation. Machine learning algorithms, trained on historical data derived from thousands of patients, can perform the following functions:
Continuous Monitoring of Vital Signs: AI consistently assesses parameters such as heart rate, blood pressure, respiratory rate, and temperature to identify early warning indicators of sepsis.
Detection of Subtle Trends: Advanced algorithms can uncover minor alterations in biomarkers or vital sign trends that may precede clinical symptoms.
Provision of Predictive Insights: AI systems can forecast the likelihood of sepsis several hours before symptoms onset, facilitating proactive treatment measures.
For instance, by integrating electronic health records (EHR) with AI technologies, healthcare institutions can implement predictive models that notify clinicians of potential sepsis cases, enabling timely intervention.
The Success Story: A 25% Reduction in Sepsis Mortality
A significant example of the influence of artificial intelligence (AI) on the detection of sepsis is demonstrated in a study published in Scientific Reports. This research illustrates how an AI-driven predictive analytics system facilitated a 25% reduction in sepsis-related mortality within the first year of its implementation at a leading hospital. The key factors contributing to this achievement included:
Comprehensive Data Integration: The AI system proficiently assimilated electronic health record (EHR) data, laboratory results, and bedside monitoring to establish a comprehensive overview of each patient.
Real-Time Alerts: Clinicians received timely notifications regarding patients at risk of sepsis, often hours before clinical symptoms became apparent.
Targeted Interventions: The system's early detection enabled the prompt administration of antibiotics and fluid resuscitation, critical components of effective sepsis management.
This case exemplifies AI's transformative potential in enhancing patient outcomes and alleviating burdens on healthcare systems, as substantiated by this study's findings (Nature Scientific Reports, 2022, DOI: 10.1038/s41598-022-16632-2).
Challenges and Future Potential
The integration of artificial intelligence (AI) in the detection of sepsis presents significant opportunities; however, it is accompanied by several challenges that must be addressed:
Data Quality and Availability: The effectiveness of AI models depends on access to high-quality and comprehensive datasets, which are not always readily available.
Integration with Clinical Workflows: Integrating AI into existing hospital systems requires meticulous planning and stakeholder collaboration.
Ethical and Regulatory Concerns: Safeguarding patient privacy and complying with regulatory requirements are critical to facilitating the widespread adoption of AI technologies.
Furthermore, advancements in wearable technology, remote monitoring, and federated learning can enhance AI's capabilities in sepsis detection. These innovations could facilitate real-time monitoring of at-risk patients outside the intensive care unit, broadening the scope of critical care delivery.
Conclusion
The integration of artificial intelligence significantly enhances sepsis detection, serving as a vital resource for patients and healthcare providers. By discerning subtle patterns in vital signs and delivering predictive insights, artificial intelligence facilitates earlier interventions and improved patient outcomes, thus fostering a more promising future for critical care. As technological advancements continue, the incorporation of artificial intelligence into clinical practice possesses the potential to save numerous lives.
For those interested in understanding how artificial intelligence transforms critical care, we invite you to subscribe to our newsletter. This resource provides the latest updates, case studies, and expert insights in the field.
Reply